November 1, 2024
Long-range traffic forecasts are used to inform the selection and design of transportation projects based on predicted demand of the roadway system decades into the future. As you can imagine, there are many hard-to-predict variables that influence what traffic demands might be in 20-30 years. Traffic forecasters have a challenging job, looking at variables like predicted population, expected job growth, and land use patterns across large regions to create as accurate a forecast as possible. For decades, these types of predictions have served as the primary tool for guiding decisions about the future our streets.
However, like all efforts to predict the future, traffic forecasting is not a foolproof science. Senior Principal Engineer and experienced travel demand modeler Mike Aronson emphasizes that a model is only as good as the inputs and assumptions that go into it. “If those assumptions are inflated, the long-range traffic forecasts very often outpace the reality of traffic growth,” says Mike. “This is not the fault of the model; it’s a byproduct of the land use assumptions.”
Associate Planner/Urban Designer Scott Curry adds to this by pointing out: “There are a variety of values-driven decision-making criteria that are more important for the safety and quality of life of real people today than estimated travel delay for hypothetical future travelers.”
Ultimately, it’s important to balance data from traffic forecasts with values-driven decision making in order to assess tradeoffs regarding the future of our streets. Journey with us to dig into the accuracy of long-range forecasts, and how to use them as one of many planning tools we have at our disposal.
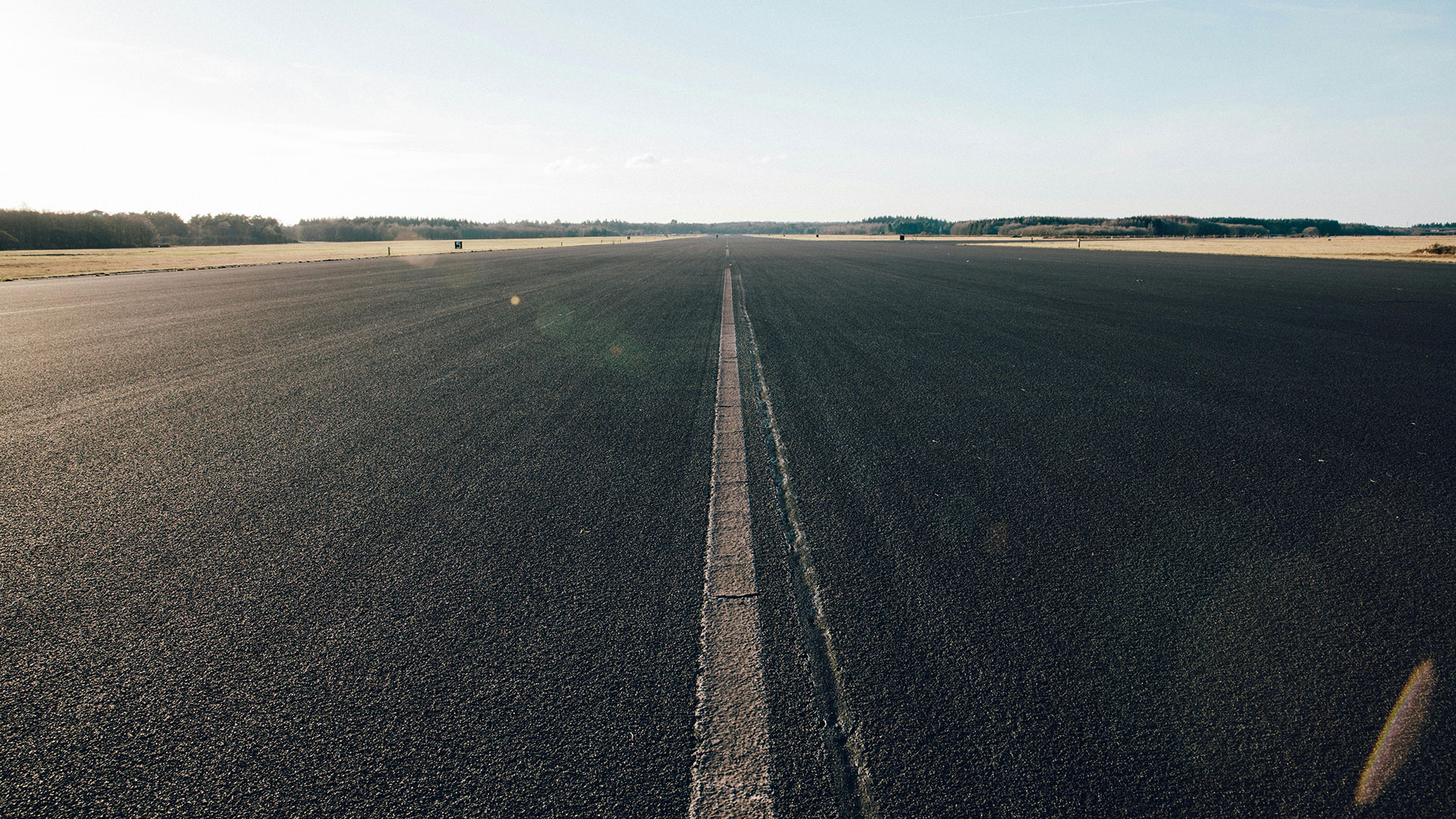
A travel demand model is only as good as the inputs and assumptions that go into it. If those assumptions are inflated, the long-range traffic forecasts very often outpace the reality of traffic growth.
How Accurate Are Long-Range Forecasts?
Despite the sophistication of today’s travel demand models, it’s simply impossible to forecast all the variables that influence future roadway demand. For example, traffic forecasts created in the year 2000 very likely did not predict the impacts of a global pandemic in 2020. Even in less extreme circumstances, human behavior is unpredictable, and the best we can do is “get close enough” to aid in decision-making. NCHRP Research Report 934, Traffic Forecasting Accuracy Assessment Research, puts it this way: “Forecasts will always be influenced by some factors that are unexpected, unpredictable, and difficult to anticipate.”
NCHRP Research Report 934 documents the work done by a research team headed by the University of Kentucky to analyze and improve the accuracy, reliability, and utility of project-level traffic forecasts. They compared traffic forecasts made at the time projects were planned with real-world traffic measured after the projects were completed, compiling a database of forecasts and subsequent traffic counts for 1,291 projects from six states and four European countries.
They found that traffic forecasts typically have a bias for overestimating future travel demand. In most cases, this bias was modest—with actual travel demand (ADT) about 6% lower than the previously predicted demand—but in some cases the overestimation bias was much more significant. It’s important to note that even a small difference between projected growth and actual growth could translate to big differences in project assumptions that guide tradeoffs. For example, a slightly over-inflated prediction could mean the difference between developing a project within current right-of-way widths, or displacing homes and businesses to accommodate road widenings that don’t turn out to be necessary.
The danger is not in using traffic forecasts in the first place; they are a useful tool that can help us align planning efforts with future regional needs. The danger happens when model outputs are taken at face value and seen as the answer for how we should make decisions about our roadways.
Understand Your Model
Kittelson’s travel demand modelers say the best way to interpret traffic forecasts is to not simply take the numbers and move on, but understand how and why a model arrived at the results it gave you, and to ensure those model results are appropriately weighed against community values and our professional imperative to support transportation equity and safety.
Model results are heavily influenced by the assumptions that go into it from the land use side—things like predicted population, jobs, and travel over a region. State agencies work with MPOs and TPOs to come up with growth projections based on land use expectations and predictions about how the area will grow into the future. Again, this is a “best guess” situation, and it tends to skew in favor of overpredicting demand. Overpredictions can be tied to a variety of factors, from over-estimating future private development, to underestimating the generational shifts that continue to erode single-occupancy vehicle (SOV) trips, such as a decline in driving age individuals getting drivers’ licenses and more people choosing to telecommute or flex commute.
The same NCHRP report dug into this, examining the common sources of forecast errors by doing a deep dive into six case studies. They found that reasons for inaccuracy were diverse, but employment, population, and fuel price forecasts frequently contribute to traffic forecast inaccuracy. Perhaps of bigger importance than the reasons for inaccuracy was the finding that for all six case studies they analyzed in detail, the traffic forecasts showed optimism bias. For each project, the observed traffic ended up being less than the forecast traffic.
“Peak Spreading” in a Post-Pandemic World
Let’s throw one more wrinkle into this: the lingering uncertainty surrounding travel patterns since the height of the COVID-19 pandemic. Changes in travel patterns from the pandemic, such as the increase in flexible or hybrid working schedules, have made it even more challenging to develop accurate long-range traffic forecasts.
One pattern that many cities are seeing is “peak-spreading”, meaning the morning and afternoon rush hours are not as severe as they used to be, since many commuters take advantage of increased flexibility to make their commute trips at different times—or to work flex schedules that only require commuting into the office 3-4 days per week. As a result, regional commute patterns are changing. These shifts provide further reason not to base design decisions on cars at the peak hour, but rather to focus on prioritizing safety and accessibility for all modes of transportation throughout the day. They also open the door for us to reallocate road space to support greater transportation equity by installing bus-only lanes, bike lanes, and/or sidewalks or sidepaths.
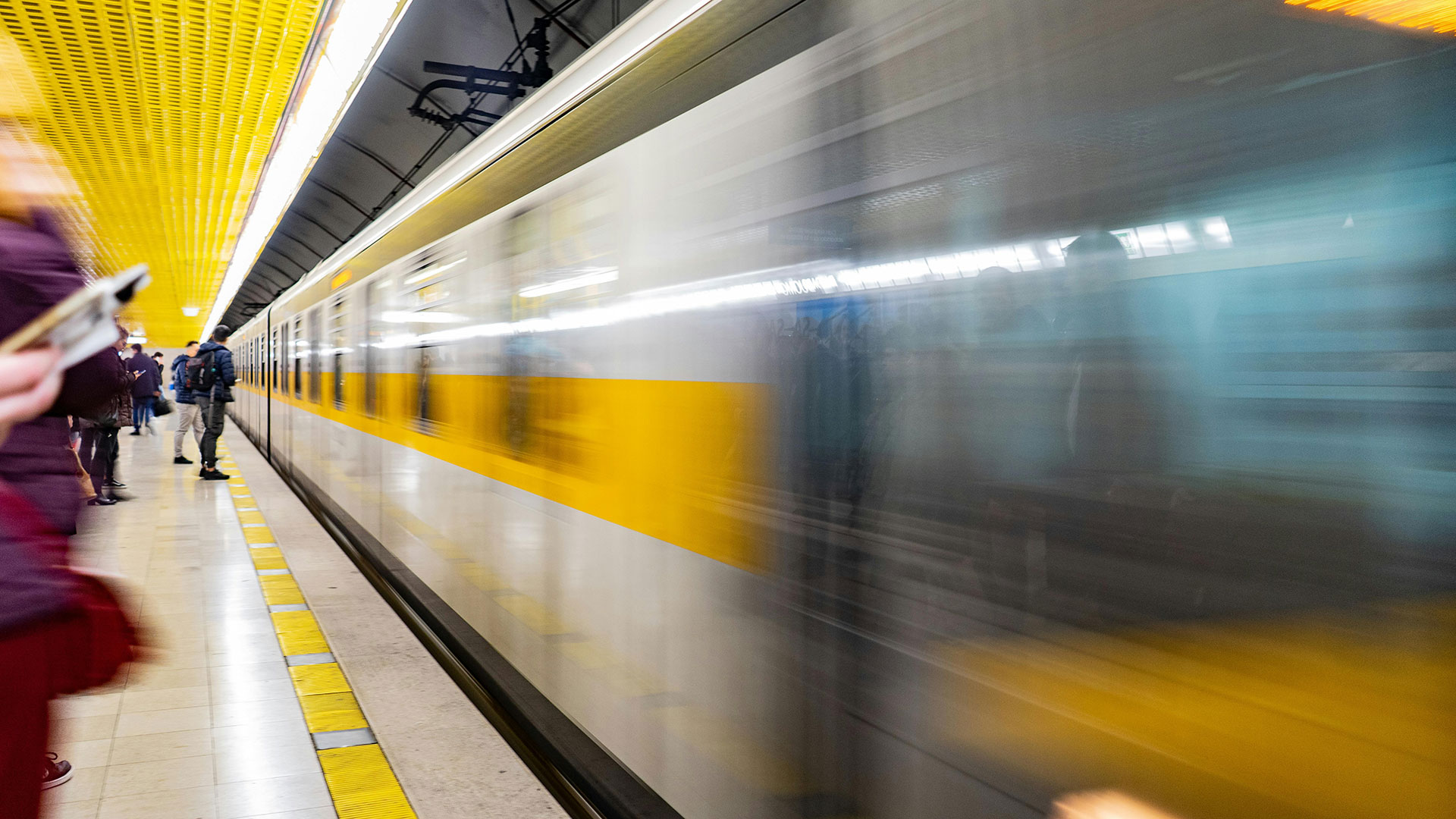
Many commuters have taken advantage of increased flexibility to make their commute trips at different times, or to travel into the office less frequently.
When you understand what inputs went into a travel demand model to get to the answer, then you know how to integrate it into your decision-making process in a balanced way. Because the reality is, there are always multiple interpretations of the data being presented. For example, you could widen a road to accommodate predicted growth, but you could also add more transit—and these decisions are best made through combining traffic forecasts with values-based considerations, like community input, supporting transportation equity, protecting vulnerable roadway users, preserving community character, and other contextual and historical information. It’s not the model’s job to figure this out; it’s the transportation planner’s job using all information available. It’s also the our responsibility as engineers and planners to understand that “the data” from a traffic forecast might not be the best decision-making tool to guide decisions about our streets.
Putting Traffic Forecasts in Their Place
In summary, traffic forecasts/models are an important tool in the toolbox for transportation engineers and planners, but their use requires an understanding of:
- the inherent limitations and inaccuracies of traffic forecasts,
- the broad assumptions that informed them, and most importantly,
- the values-driven priorities of real human beings, which may be more important than the estimated potential travel delay of hypothetical future travelers.
As with many other aspects of transportation engineering and planning, cold adherence to “the data” keeps us from seeing the whole story. Our job as planners and engineers is to make decisions driven by both data and values, so that our transportation networks grow in step with our communities and their needs, both today and in the future.
—
Bust more transportation myths with us! You can also read about: