January 28, 2019
Machine learning has been an important tool for several decades. It has been used for diverse tasks such as handwriting recognition, patient monitoring, facial recognition, and diagnoses of health conditions. Retail and financial services industries use predictive analytic tools based on machine learning to better target specific market segments.
The transportation industry is just beginning to use machine learning and big data in new and innovative ways. Transportation planning often involves making large decisions based on a variety of available data sources. Machine learning offers a vast array of tools to analyze these diverse data sets in a unified fashion. For example, structured datasets on commuter patterns provides information on the average travel distance to work. These data inform planners on how people move around their cities, and they can help us make those commutes more efficient.
Machine Learning in Transportation Data Analytics
What makes data “big” is not necessarily the amount of data – it is the predictive measures built upon it that allow us to make more informed decisions. If new public transportation infrastructure is built, how many commuters will use it? Which neighborhoods will benefit most? As autonomous vehicle technology improves, will public transportation use decline?
Big data and machine learning can help transportation planners and engineers find the answers to complex transportation questions.
Approximately 44 percent of drivers use smartphone apps for navigation, creating valuable data for urban planners and transit agencies to answer some of those questions. Instead of relying on random samples or lengthy surveys, transportation engineers and analysts can derive valuable insights from existing transit monitoring equipment and/or passenger data from a transit app or other resources.
With thousands of individual journeys to analyze, developing insights from raw data can be challenging. Here are a few examples of how researchers and municipalities are using machine learning to use big data to develop effective transportation solutions.
Machine Learning in Transportation
A tool built by the University of Texas at Austin scans footage from existing traffic cameras to automatically analyze road activity. Using deep learning, the system can identify objects such as bicycles, buses, and pedestrians, and automatically create searchable databases that helps planners understand road use. Researchers use the pre-sorted data to identify where collisions and close encounters occurred between vehicles and pedestrians and prioritize these areas for safety enhancements.
Further down I-35 in San Antonio, traffic signals coordinate with each other to create the most efficient interval based upon real-time traffic density. Since installing these “smart” signals, throughput has increased up to 60 percent and more than 8.6 motorist hours have been saved.
Machine learning can play an important role in improving travel forecasting and traffic flow prediction. Our current models are too limited; they rely on restrictive assumptions that seldom hold in practice, and they cannot capture the complexities involved in travel behavior. We need to consider how new technology can complement existing public transportation and lead to more effective use of public dollars for future infrastructure projects. In Los Angeles, a city with notoriously bad traffic, researchers at USC’s Viterbi School of Engineering used machine learning to develop a speed-forecasting method that is 12 to 15 percent more accurate than traditional, statistical methods. According to a USC press release, the method uses both historical and real-time data to predict future speeds along a road. The system improves over time by learning which methods predict the most accurate speeds.
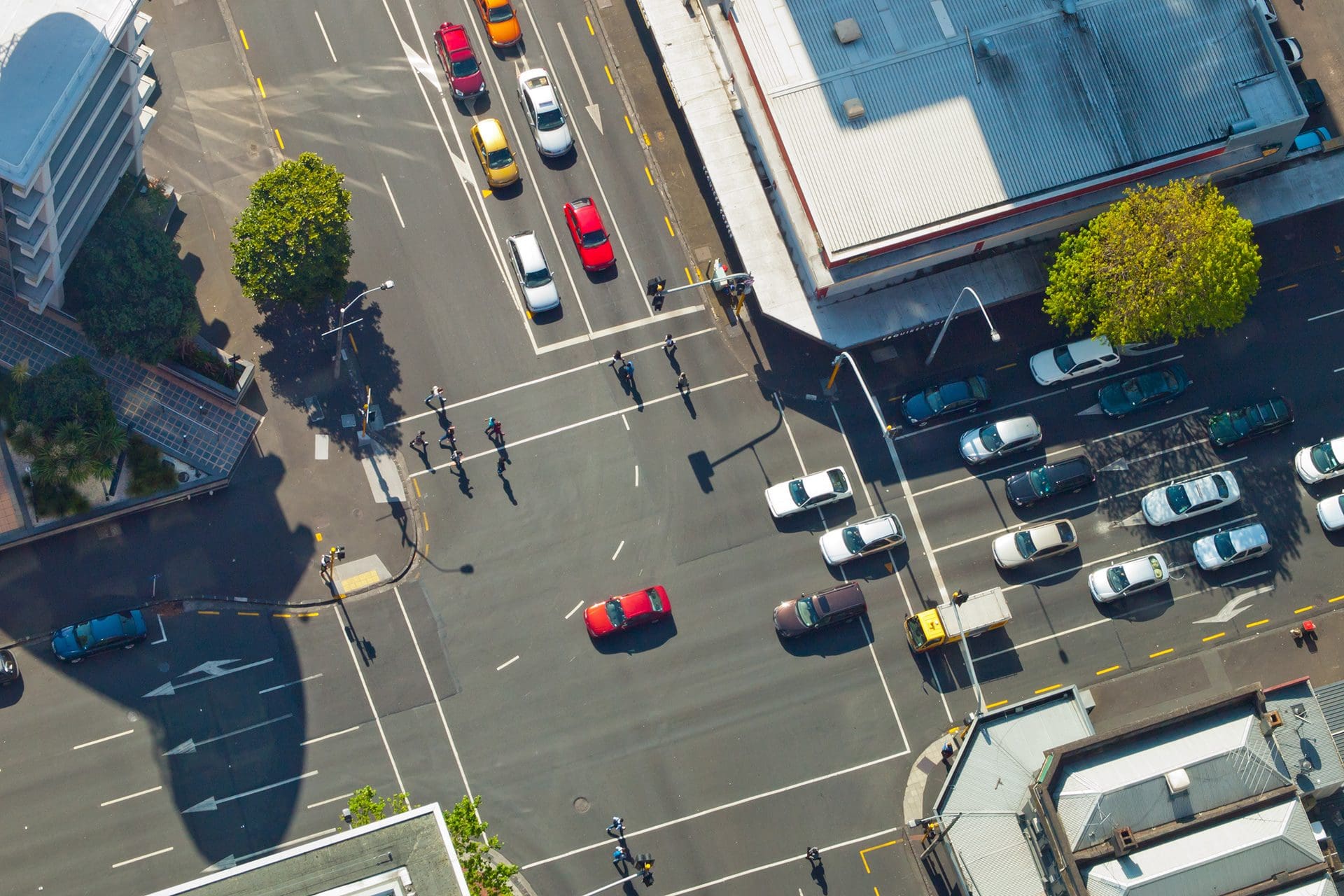
We need to consider how new transportation technologies can complement existing public transportation and lead to more effective use of public dollars for future infrastructure projects.
Machine Learning and Land Use
Machine learning can help transportation engineers and planners evaluate the potential of new technology to make informed decisions about how to adapt their local infrastructure.
New types of transportation mobility are on the horizon, from increased micro mobility options to the possibility of an automated vehicle future, affecting how we travel from place to place and how we use land. Interpreting data is essential for us to understand the best way to design spaces to meet a community’s needs.
For example, data shapes how we think about curbside design and management. In cities like Washington, D.C., data determines parking pricing, reducing congestion and addressing the city’s high parking demand. As patterns change and passengers increasingly seek out alternative forms of transportation, space used for parking today may be more valuable in the future if converted into passenger loading zones, wider sidewalks and or protected bike lanes. Data collected from ride-sharing companies includes popular pick-up and drop-off points to help cities evaluate and adapt to the changing transportation needs of their local population.
The specifics of how new technologies will change infrastructure needs may differ by city, but planners can expect some recurring patterns. For example, as cities adopted ridesharing services such as Uber and Lyft, ambulance requests declined by 7 percent. Big data will also be key in determining the effect of automated vehicles in early-adopter cities.
By tracking and sharing data on the effect of automated vehicles in early-adopter cities, transportation planners and engineers can better prepare for their roll out nationwide.
Transportation Research Board Activities
The Transportation Research Board Committee on Artificial Intelligence and Advanced Computing Applications (ABJ70) was created to promote the use of artificial intelligence and machine learning methods in transportation. One of the most important activities of this committee is conducting outreach among transportation professionals to inform them of the potential benefits of machine learning methods.
The committee conducted a workshop on big data and machine learning at the TRB 2019 Annual Meeting, directed at practitioners who may have had little or no familiarity with machine learning. The workshop included an overview of machine learning essentials, an overview of several important machine learning methods, and a review of the necessary background for machine learning. The workshop included presentations from the five finalists of the TRANSFOR19 Data Competition, sponsored by IEEE and DiDi. The workshop was well attended, demonstrating practitioners’ interest in learning more about the capabilities of machine learning methods to revolutionize transportation analysis.
The ABJ70 committee is now developing a primer on machine learning and transportation. Targeted at practicing professionals, the primer is scheduled to be issued in the latter half of 2019.
The Future of Machine Learning and Transportation Engineering
As vehicles get smarter and data get bigger, machine learning will be an increasingly important tool for transportation planners and engineers. With that in mind, we need to continue to inform transportation professionals about machine learning.
When transportation planners and engineers can use machine learning to analyze big data, cities can quickly and efficiently identify trends and detect patterns to make data-driven decisions about future infrastructure and spending. Ten years from now, predictive analytics may enable transportation professionals to counteract congestion before it happens, deploy micro-routes on the fly and use smart transit technology to cut costs while improving service and safety.