August 7, 2024
The term “artificial intelligence” (AI) has become a part of the everyday vocabulary in part because it has become a much bigger part of our everyday lives. The proliferation of commonplace AI technologies and applications, like the 2022 arrival of OpenAI’s ChatGPT, has sparked no shortage of conversation, disagreement, and the occasional doomcast regarding the future of work and the world.
In transportation, AI-powered connected and autonomous vehicles (CAVs) have been an emerging transportation technology and topic of debate in transportation discourse for even longer. WAYMO, the self-driving vehicle technology firm that originated as a Google passion project, first began offering fully autonomous rideshare services in the Phoenix Metro area in 2018; in the intervening years the company has expanded service to San Francisco and Los Angeles. Elsewhere, some young planned communities have built their transit systems using fleets of AI-powered vehicles. Other commercial car companies have attempted to capitalize on consumer appetite for CAVs, sometimes with results that seem to confirm AI doomsayers’ worst fears.
Does the participation of AI on our roadways make transportation safer or more dangerous? This question requires us to put aside the preconceptions we have of AI – whether as a friendly neighborhood chatbot ready to generate midweek meal suggestions for the busy professional, or as a Kubrickian agent of dispassionate megalomania, awaiting its chance to jettison the unsuspecting user into outer space – and ask ourselves how AI works. And how we do.
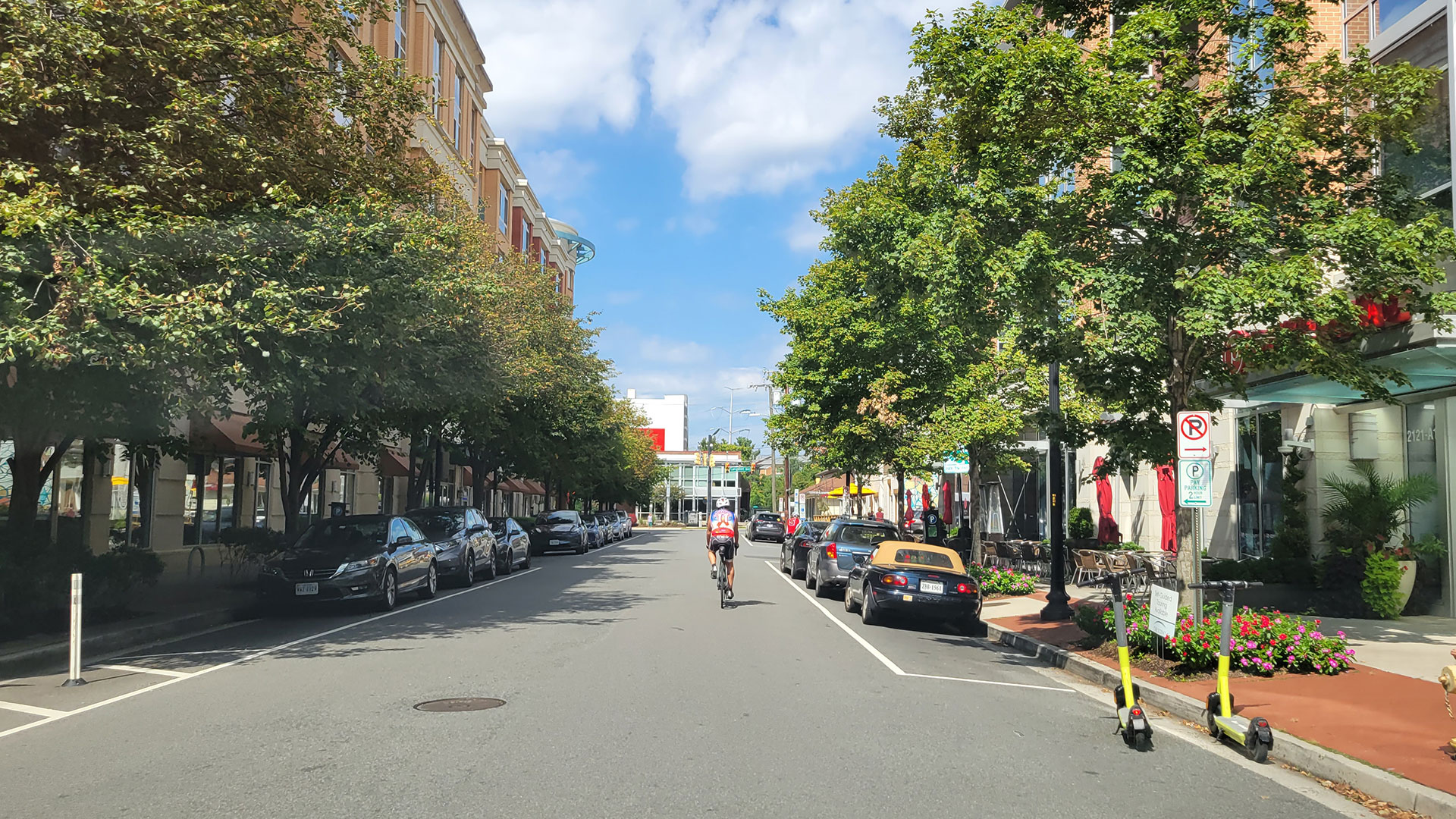
AI can outperform human analysis in specific tasks; however, it has a fundamental limitation that will keep it out of the driver's seat of transportation planning projects.
What We Talk About When We Talk About AI
First off: What do we mean when we say “AI”? For the purposes of our discussion, we’ll define AI not by any of its individual applications (or appearances in pop culture) but rather by the way it works. When we talk about “AI,” we’re talking primarily of advanced mathematical applications of machine learning to perform pattern recognition. In the transportation industry, large-scale, sophisticated machine-learning can be applied to process large amounts of visual information or other transportation data to do a range of tasks beyond CAVs, from identifying where and when to make repairs to transportation facilities to evaluating crash history and roadway conditions to develop more proactive approaches to improving safety.
Interestingly, the research, development, and commercialization of AI-powered CAVs and other AI applications have occurred largely independently of the public sector. While much of the work can be tied back to academic research, more recent application has been driven by private companies. In response, state DOTs and local agencies have looked to gain greater understanding of AI, evaluated how to “futureproof” transportation systems, and had many, many conversations at TRB. However, the transportation profession faces substantial obstacles when choosing how to use and engage with AI applications:
- Missing skillsets: Planners and engineers are experts at planning and engineering – not at understanding how to design or work with AI. Employing a computer scientist capable of writing and maintaining an AI model may seem like an unrealistic or off-mission luxury to a transportation agency. Conversely, private-sector technology developers may have critical blind spots regarding the complexities of the transportation system or face incentive structures (profit / proof of technology) that are misaligned with agency goals (safety / public comfort).
- High upfront cost: Even if a jurisdiction has a dedicated staff capable of developing an AI powered model, there are substantial time and computer costs that make the work prohibitively expensive. Once established a trained AI model can process a huge amount of data at a fraction of the time it would take a human analyst to do so, but to get to that point a human analyst must spend hours and hours sourcing and curating data. Private vendors with multiple clients or deep pocketed investors have greater ability to absorb these upfront costs; however, agency staff are often still responsible for preparing and providing data and validating outputs.
- Absence of established record: Currently, AI applications are still in their infancy, with many vendors that sell AI powered applications only a year or two old with few existing clients. There is massive potential for these technologies. But at this point it is still up to transportation professionals to balance this promise with questions about relative benefits compared to existing approaches, the ability to check outputs and outcomes, and understand real risk to people in the transportation system.
However, perhaps the biggest problem with using AI in safety planning extends beyond any locality’s practical limitations in harnessing the technology and to a fundamental incompatibility of how transportation planners and machine learning conduct “thought.”
What keeps AI out of the driver's seat of transportation planning projects is a fundamental incompatibility of how transportation planners and machine learning conduct “thought.”
AI’s Struggle to Understand Causality
While machine learning models can find patterns in an enormous number of factors much faster than a human can, they have difficulty setting these factors in context or weighting them per relevance. This is because models don’t reason through factors so much as they guess (or, more generously, predict) about them based on how those factors have functioned in the data they were trained on. This can lead to a model drawing only superficially correct – or, in some cases, wildly incorrect – false-causations, like determining that daylight is the cause of crashes at a high-risk intersection simply because most of the data set’s crashes occurred during the day. To understand how this can be problematic for the transportation profession, let’s consider the issues of causality and data sources.
Causality is the cornerstone of all roadway safety projects. When proposing engineering countermeasures as part of a Safety Action Plan or other roadway safety initiative, you need to first identify both the locations that pose high crash risk and which design feature (or features) at each high-risk location is most probably creating the safety risk. AI powered evaluations have the potential to identify characteristics that are associated with increased risk, helping to predict where crashes might occur, but improving safety requires identifying a causal relationship and a lever that can be pulled to reduce risk. Explaining the “why” is also critical for effectively communicating with decision-makers and the public as to why changes are proposed.
AI powered evaluations have the potential to identify characteristics that are associated with increased risk, helping to predict where crashes might occur, but improving safety requires identifying a causal relationship and a lever that can be pulled to reduce risk.
Similarly, AI’s need for massive datasets can result in incorporating information that is imprecise or inaccurate leading to logical, but incorrect outputs. This issue can be most pronounced in technical work where there are fewer uses of specific terms, or where technical definitions defer from common language uses in real and meaningful ways. In the most extreme cases, this could result in a chatbot like ChatGPT breaking up vocabulary that should be treated as a single unit of meaning – “roundabout,” for example – into the two discrete tokens “round” and “about,” which creates an opening for the model to devolve into a grade-school conversation about shapes. Perhaps more concerning, an unsuspecting user could look for information about specific design guidance and get an answer drawing from a range of non-technical description and statements.
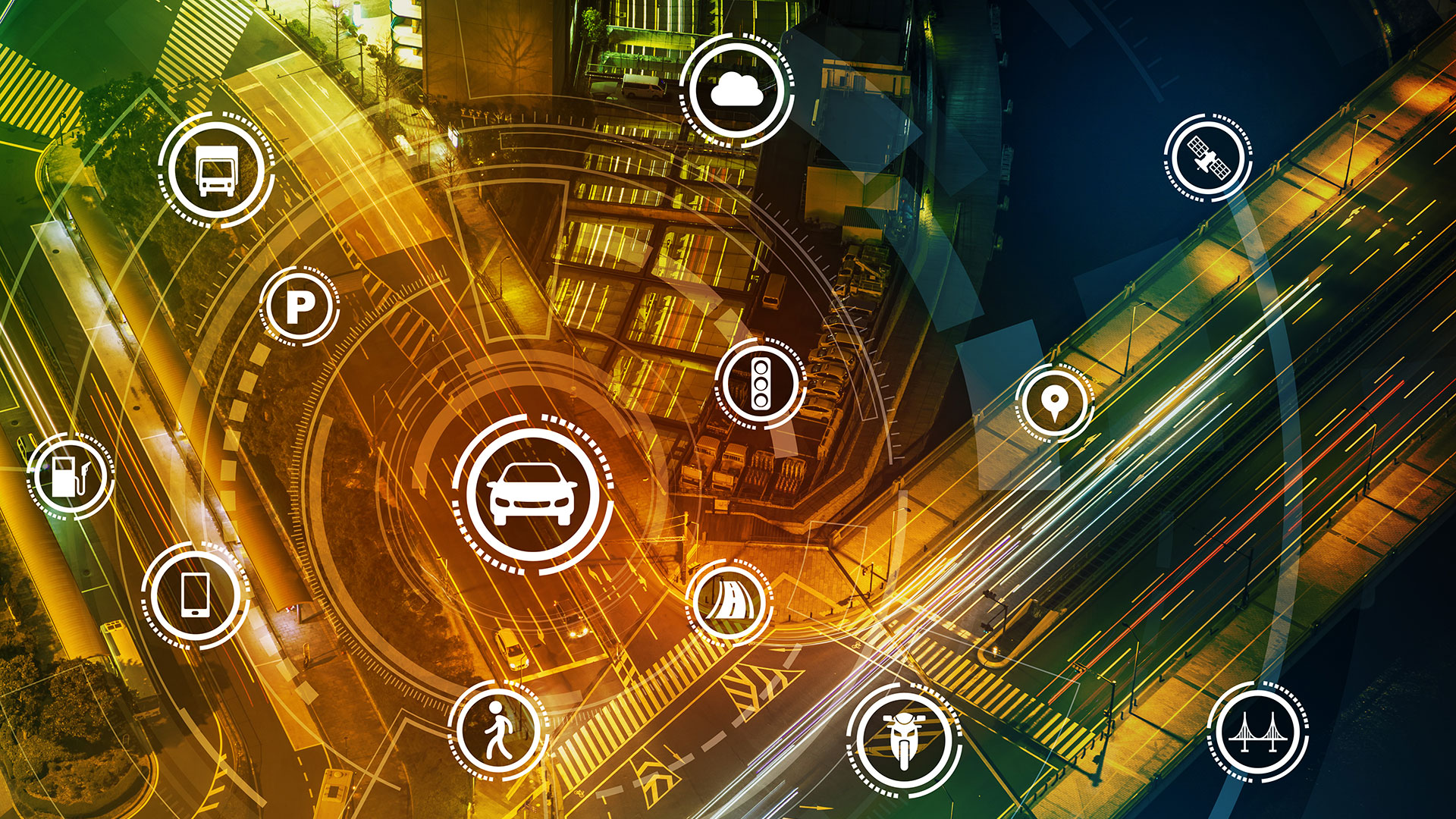
While machine learning models can find patterns much faster than a human can, they have difficulty setting these factors in context. This is because models don’t reason through factors so much as they make predictions based on how those factors have functioned in the data they were trained on.
Where AI Outperforms Human Analysis in Transportation Planning
We raise these issues not to dismiss the capabilities of AI, but to give context to where it has the greatest potential in our profession. While AI may misidentify causal relationships between situational factors, it has the potential to assist on transportation safety problems where knowing an exact cause isn’t essential. Consider, for instance, how AI could proactively position first-responders throughout a large area to minimize response time in a roadway emergency. Even if AI can’t determine exactly why crashes occur, it can assess correlation between incidence of crashes and other variables (like income, historical underinvestment, and other health factors), and then triangulate areas that possess the greatest number of those variables (and therefore possess a profile of compounded risks). By crunching a huge number of factors in seconds to determine the placement of first-response vehicles, machine learning arrives at a potentially lifesaving answer much faster than any human analyst could.
We’ve also been interested in AI’s potential to fill in missing data that can help us better understand our roadways at a fundamental level. State and local agency can struggle to maintain complete information about roadways and intersections, often missing factors such as the location of on-street parking or roadway width, and even basic information about intersections. As part of a Small Business Innovation Research grant, we recently proposed using AI to process aerial images of intersections for their characteristics to help us measure risk to people biking. Currently, the absence of quality data about intersections makes systemic evaluations of bike experience difficult, even though the majority of vehicle-bicycle crashes occur at them.
Where Do We Go from Here?
The recent proliferation and popularization of AI technologies represents an important augmentation to roadway safety planning but stops short of fully reinventing it. Although powerful, AI is not yet at a point where it can account for the intricacies and contradictions created by the behaviors of humans in motion, and accounting for these behaviors is so much of the art and the science of safety planning and engineering. We feel comfortable rejecting catastrophic imaginings of AI fully supplanting human planners and engineers, because right now the type of analysis performed by these entities is so different that extensive use of AI in safety planning would create redundant work instead of expediting it.
Instead of relying on AI as a one-stop shop for transportation planning output, think about it as the latest among many sophisticated tools that, when deployed appropriately, can strengthen our practice. Instead of turning to AI to automate steps out of the safety planning process, we advocate for thinking of AI as a tool that, depending on a project’s time, budget, and staff, might be helpful in producing data that would be hard to create in other ways and insights that regular statistics can’t capture. Whether tracking vehicles at intersections, or building additional safety data by compiling video footage of near-misses instead of outright crashes, we see AI as an exciting frontier that provides a fuller picture of the risks of our roadways; a partner in safety, not a boss.